1. Replacement of old systems and technologies
We are in the year 2024, which means that many IT technologies that were once modern and state of the art are no longer. The problem is that quite a few companies are struggling to accept this fact. There are many reasons for this: because they can't or don't want to see it, because the tried and tested seems too familiar and the new seems too new - or it simply costs too much.
It is important for organizations to recognize that the lifecycle of old technologies - especially common integration platforms - is coming to an end and needs to be replaced in a timely manner. The best example: many people who can operate these platforms will soon be retiring. There is a general lack of young talent - or they are working with completely different solutions. A generational change in the technology should take place at the latest with the generational change in the people working with it. In addition, the high license costs for outdated products are theoretically avoidable.
In short: even if it sometimes seems otherwise at first glance, it can be economically and humanly effective to replace the existing integration platform with a modern, Kafka-based approach.
2. Transition from isolated data streams to company-wide data usage
Another key challenge in this process is the transition from fragmented data streams to comprehensive, company-wide data usage. Due to historically grown data structures, companies often lack both insight and an overview of their existing data. Without this, however, it is not possible to view data holistically - let alone develop a company-wide data strategy. As a result, data is distributed almost randomly throughout the company, even in supposedly progressive sectors such as finance and insurance. This in turn means that some departments are unaware of this data, even though the information it contains would be useful for daily business.
These are not isolated cases, but an almost global problem. The transition from these uncontrolled data streams to democratized company-wide data usage is therefore one of the core tasks of modern data management. We can only speak of a smart data strategy if the people involved have access to all essential data - at any time and without restrictions within the defined scope of action.
3. Rethinking batch processing in favor of real-time data streams
At the same time, there needs to be a fundamental rethink in data processing towards the use of real-time data streams instead of traditional batch processing. Experience in recent years has shown that although tools exist for this, they are not being used correctly. It is not enough to simply provide technologies. Their use must also be anchored in the corporate culture. After all, a good tool is only effective in everyday life if it is used correctly. A good example is the leap from time-delayed batch to real-time data processing. If data is received in real-time data streams, but employees still only check the data twice a day, as is the case after batch updates, real-time data loses its greatest advantage.
It is therefore essential for companies to provide specialists with targeted training in data-related skills. In this way, the full potential of data streaming can be exploited: For example, instant payment in the financial sector or dynamic price adjustments in e-commerce would simply not be possible without real-time data. And it is precisely innovations like these that show how crucial it is to exploit the full potential of real-time processing and take advantage of the associated opportunities.
4. Governance and self-service for data products
Furthermore, companies must learn to see data as a valuable product that must be actively maintained and managed to achieve its full potential. Although most organizations are aware that data is a valuable corporate resource, data does not automatically become useful information. It only brings real benefits when it is used in the right way. To do this, it must be maintained and developed, rather like a garden. Only by carefully managing the entire data life cycle – from collection to processing to archiving – can it reach its full potential and create real added value. Without this care, data remains just raw information without much use. It is essential that a) a clearly defined data product exists and b) that it is available to all relevant teams.
The challenge is for companies to understand data as a product and use it accordingly. Take the practical example of bank customer master data: currently, this is often copied overnight into different databases to ensure that all the necessary teams have access. However, how much more efficient would it be if this process were redundant? If there were a central data product that everyone could access directly as soon as it was needed? This is precisely the goal of modern architecture teams – but to achieve it, they need the right systems, such as Kafka.
5. Organizational transformation
However, this progress can only be successfully implemented if we recognize the challenges for what they actually are: a comprehensive transformation. A central aspect here is the adaptation of responsibilities in data integration. Instead of a central data team that controls all processes and thus easily becomes a bottleneck, autonomous teams should be established that are responsible for their own data. Such teams can then flexibly access the data and process it as needed.
Kafka provides the ideal basis for this. It not only enables data processing to be highly up-to-date, but also ensures a robust and resilient system structure. If a team incorporates errors into its data products, these remain localized and do not spread to other areas like a domino effect. This keeps the overall system stable, even if problems occur in individual areas.

Webinar “From Kafka Clusters to Data Mesh”
This article only briefly touches on the challenges and solutions. The deep dive will be provided in a free webinar from 2:00 to 2:45 p.m. on September 26 (German only).
Frequently asked questions
All blog posts
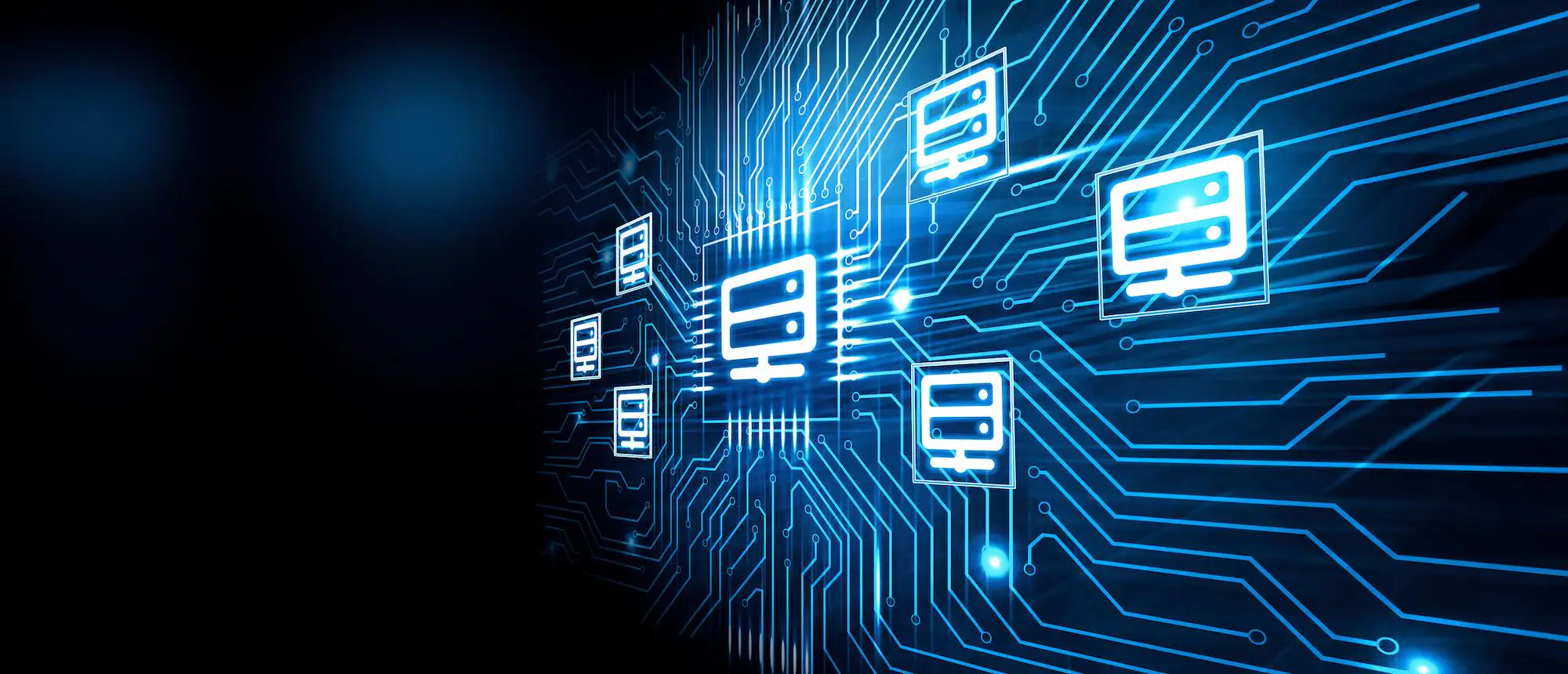
Apache Kafka simply explained
In today’s world, where data needs to be processed faster and in ever-increasing volumes, a reliable and scalable infrastructure is essential. Apache Kafka has emerged as a leading solution for real-time data streaming and is used by businesses worldwide to capture, analyze, and distribute data efficiently. In this blog post, we explain what Apache Kafka is, how it works, and why it is crucial for modern enterprises—simply and clearly.

Good data management is the basis for the business models of tomorrow
The rapid spread of artificial intelligence also creates new challenges for companies when it comes to data. Dirk Budke, Lead Data Engineering & AI at mesoneer, explains the importance of strategic data management and why employers should proactively introduce AI tools.

The age of AI offers many opportunities - also for SMEs
The relevance of digital technologies is increasing, both in a professional and private context. mesoneer AG therefore operates right at the interface between people and technology - and uses its products and software solutions to ensure smooth interaction between companies and customers while maximizing added value. In this interview, Dirk Budke, Lead Data Engineering & AI at mesoneer, explains exactly how this works.